A question about whether Republican vice presidential candidate Sen. JD Vance (R-OH) would challenge the 2024 election results quickly devolved into a fight about censorship and Big Tech during the debate with Democratic candidate Gov. Tim Walz (D-MN).
Technology
Save $40 on the Ninja Creami ice cream maker with this Prime Day deal
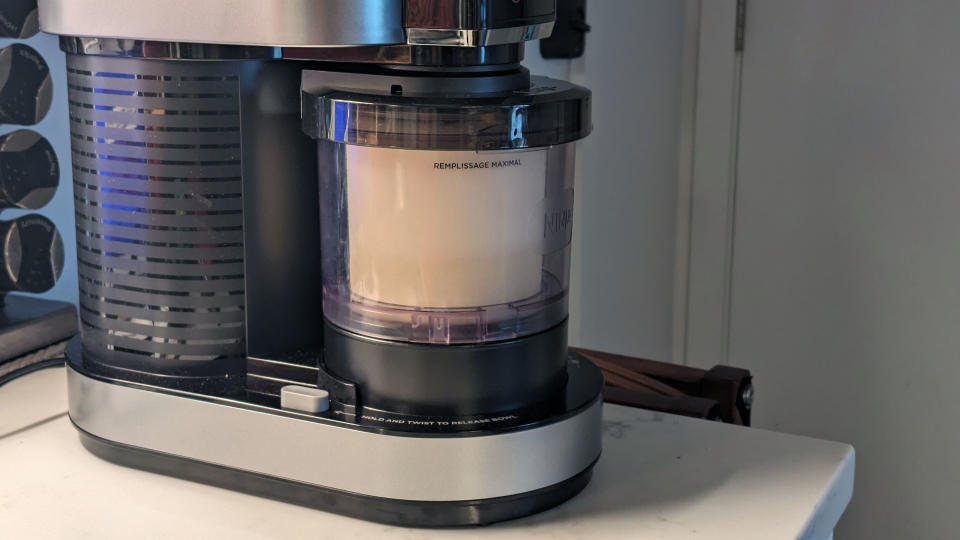
An early Amazon Prime Day deal lets you save $40 on the Ninja Creami Deluxe ice cream maker. The 11-in-1 kitchen accessory, one of our favorite kitchen gadgets, is on sale for only $210 (usually $250).
The Creami is based on a patent for an innovation previously only found in the restaurant biz. Once it expired, Ninja leaped at the chance to incorporate its design into a relatively affordable consumer product. The result is fast, easy and surprisingly yummy ice cream made from home.
Instead of the churning method used in most ice cream makers, the Ninja Creami uses a drill-like utensil to blend a frozen liquid base into a tasty dessert. Although it’s pretty loud while doing its thing, it’s still quieter than most countertop blenders — and only stays noisy for a few minutes.
When reviewing the standard Creami model (this Deluxe one includes extra containers and settings), Engadget’s Sam Rutherford concluded that tasting its resulting ice cream was “the best part.” It produces desserts with intense flavors and smooth textures. This higher-end model also makes smoothies and Frappuccino-style coffee drinks.
“Because the base is spun instead of churned, there’s less air inside your finished product,” our reviewer wrote. “This is good because it increases flavor intensity and delivers a slightly denser, more luxurious mouthfeel. It’s almost closer to a frozen custard than ice cream.”
You can get the Ninja Creami Deluxe right now on Amazon for $210.
Follow @EngadgetDeals on Twitter for the latest tech deals and buying advice in the lead up to October Prime Day 2024.
Technology
Women of color running for Congress are attacked disproportionately on X, report finds
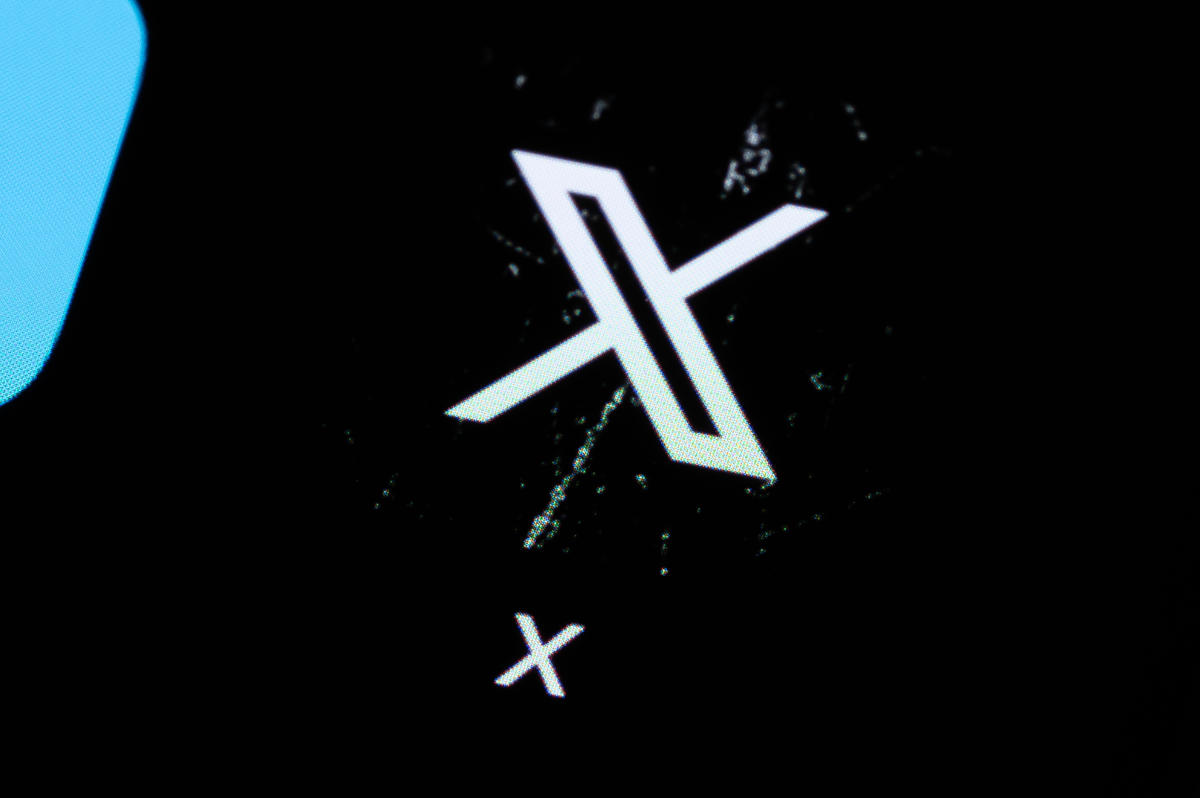
Women of color running for Congress in 2024 have faced a disproportionate number of attacks on X compared with other candidates, according to a new report from the nonprofit Center for Democracy and Technology (CDT) and the University of Pittsburgh.
The report sought to “compare the levels of offensive speech and hate speech that different groups of Congressional candidates are targeted with based on race and gender, with a particular emphasis on women of color.” To do this, the report’s authors analyzed 800,000 tweets that covered a three-month period between May 20 and August 23 of this year. That dataset represented all posts mentioning a candidate running for Congress with an account on X.
The report’s authors found that more than 20 percent of posts directed at Black and Asian women candidates “contained offensive language about the candidate.” It also found that Black women in particular were targeted with hate speech more often compared with other candidates.
“On average, less than 1% of all tweets that mentioned a candidate contained hate speech,” the report says. “However, we found that African-American women candidates were more likely than any other candidate to be subject to this type of post (4%).” That roughly lines up with X’s recent transparency report — the since Elon Musk took over the company — which said that rule-breaking content accounts for less than 1 percent of all posts on its platform.
Notably, the CDT’s report analyzed both hate speech — which ostensibly violates X’s policies — and “offensive speech,” which the report defined as “words or phrases that demean, threaten, insult, or ridicule a candidate.” While the latter category may not be against X’s rules, the report notes that the volume of suck attacks could still deter women of color from running for office. It recommends that X and other platforms take “specific measures” to counteract such effects.
“This should include clear policies that prohibit attacks against someone based on race or gender, greater transparency into how their systems address these types of attacks, better reporting tools and means for accountability, regular risk assessments with an emphasis on race and gender, and privacy preserving mechanisms for independent researchers to conduct studies using their data. The consequences of the status-quo where women of color candidates are targeted with significant attacks online at much higher rates than other candidates creates an immense barrier to creating a truly inclusive democracy.”
Technology
DeepMind’s SCoRe shows LLMs can use their internal knowledge to correct their mistakes
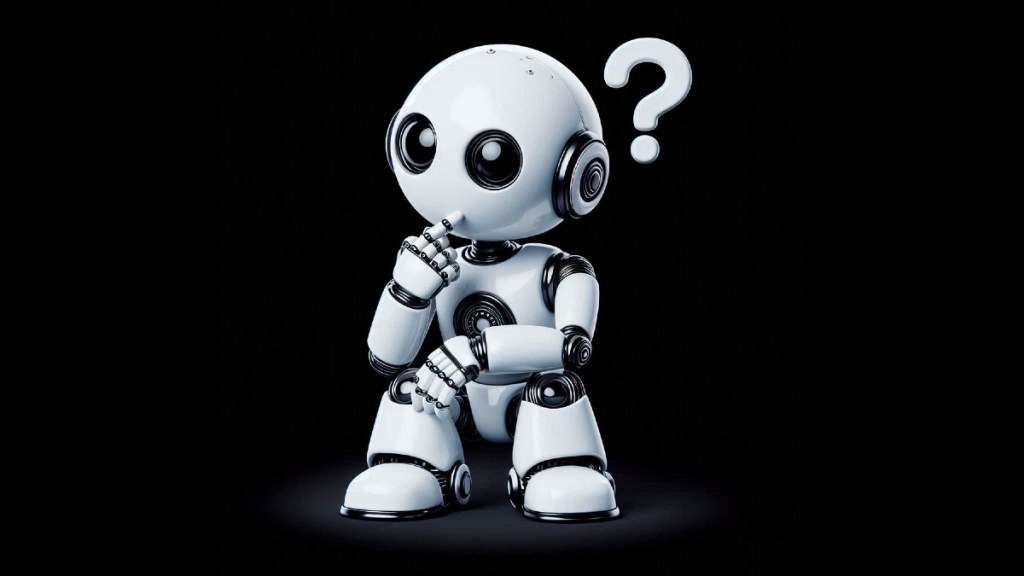
Join our daily and weekly newsletters for the latest updates and exclusive content on industry-leading AI coverage. Learn More
While large language models (LLMs) are becoming increasingly effective at complicated tasks, there are many cases where they can’t get the correct answer on the first try. This is why there is growing interest in enabling LLMs to spot and correct their mistakes, also known as “self-correction.” However, current attempts at self-correction are limited and have requirements that often cannot be met in real-world situations.
In a new paper, researchers at Google DeepMind introduce Self-Correction via Reinforcement Learning (SCoRe), a novel technique that significantly improves the self-correction capabilities of LLMs using only self-generated data. SCoRe can be a valuable tool for making LLMs more robust and reliable and opens new possibilities for enhancing their reasoning and problem-solving abilities.
The importance of self-correction in LLMs
“Self-correction is a capability that greatly enhances human thinking,” Aviral Kumar, research scientist at Google DeepMind, told VentureBeat. “Humans often spend more time thinking, trying out multiple ideas, correcting their mistakes, to finally then solve a given challenging question, as opposed to simply in one-shot producing solutions for challenging questions. We would want LLMs to be able to do the same.”
Ideally, an LLM with strong self-correction capabilities should be able to review and refine its own answers until it reaches the correct response. This is especially important because LLMs often possess the knowledge needed to solve a problem internally but fail to use it effectively when generating their initial response.
“From a fundamental ML point of view, no LLM is expected to solve hard problems all within zero-shot using its memory (no human certainly can do this), and hence we want LLMs to spend more thinking computation and correct themselves to succeed on hard problems,” Kumar said.
Previous attempts at enabling self-correction in LLMs have relied on prompt engineering or fine-tuning models specifically for self-correction. These methods usually assume that the model can receive external feedback on the quality of the outputs or has access to an “oracle” that can guide the self-correction process.
These techniques fail to use the intrinsic self-correction capabilities of the model. Supervised fine-tuning (SFT) methods, which involve training a model to fix the mistakes of a base model, have also shown limitations. They often require oracle feedback from human annotators or stronger models and do not rely on the model’s own knowledge. Some SFT methods even require multiple models during inference to verify and refine the answer, which makes it difficult to deploy and use them.
Additionally, DeepMind’s research shows that while SFT methods can improve a model’s initial responses, they do not perform well when the model needs to revise its answers over multiple steps, which is often the case with complicated problems.
“It might very well happen that by the end of training the model will know how to fix the base model’s mistakes but might not have enough capabilities to detect its own mistakes,” Kumar said.
Another challenge with SFT is that it can lead to unintended behavior, such as the model learning to produce the best answer in the first attempt and not changing it in subsequent steps, even if it’s incorrect.
“We found behavior of SFT trained models largely collapses to this ‘direct’ strategy as opposed to learning how to self-correct,” Kumar said.
Self-correction through reinforcement learning

To overcome the limitations of previous approaches, the DeepMind researchers turned to reinforcement learning (RL).
“LLMs today cannot do [self-correction], as is evident from prior studies that evaluate self-correction. This is a fundamental issue,” Kumar said. “LLMs are not trained to look back and introspect their mistakes, they are trained to produce the best response given a question. Hence, we started building methods for self-correction.”
SCoRe trains a single model to both generate responses and correct its own errors without relying on external feedback. Importantly, SCoRe achieves this by training the model entirely on self-generated data, eliminating the need for external knowledge.
Previous attempts to use RL for self-correction have mostly relied on single-turn interactions, which can lead to undesirable outcomes, such as the model focusing solely on the final answer and ignoring the intermediate steps that guide self-correction.
“We do see… ‘behavior collapse’ in LLMs trained to do self-correction with naive RL. It learned to simply ignore the instruction to self-correct and produce the best response out of its memory, in zero-shot, without learning to correct itself,” Kumar said.
To prevent behavior collapse, SCoRe uses a two-stage training process with regularization techniques. The first stage replaces SFT with a process that optimizes correction performance while ensuring that the model’s initial attempts remain close to the base model’s outputs.
The second stage employs multi-turn RL to optimize reward at both the initial and subsequent attempts while incorporating a reward bonus that encourages the model to improve its responses from the first to the second attempt.
“Both the initialization and the reward bonus ensure that the model cannot simply learn to produce the best first-attempt response and only minorly edit it,” the researchers write. “Overall, SCoRe is able to elicit knowledge from the base model to enable positive self-correction.”
SCoRe in action
The DeepMind researchers evaluated SCoRe against existing methods that use self-generated data for self-correction training. They focused on math and coding tasks, using benchmarks such as MATH, MBPP, and HumanEval.

The results showed that SCoRe significantly improved the self-correction capabilities of Gemini 1.0 Pro and 1.5 Flash models. For example, SCoRe achieved a 15.6% absolute gain in self-correction on the MATH benchmark and a 9.1% gain on the HumanEval benchmark in comparison to the base model, beating other self-correction methods by several percentage points.
The most notable improvement was in the model’s ability to correct its mistakes from the first to the second attempt. SCoRe also considerably reduced the instances where the model mistakenly changed a correct answer to an incorrect one, indicating that it learned to apply corrections only when necessary.
Furthermore, SCoRe proved to be highly efficient when combined with inference-time scaling strategies such as self-consistency. By splitting the same inference budget across multiple rounds of correction, SCoRe enabled further performance gains.

While the paper primarily focuses on coding and reasoning tasks, the researchers believe that SCoRe can be beneficial for other applications as well.
“You could imagine teaching models to look back at their outputs that might potentially be unsafe and improve them all by themselves, before showing it to the user,” Kumar said.
The researchers believe that their work has broader implications for training LLMs and highlights the importance of teaching models how to reason and correct themselves rather than simply mapping inputs to outputs.
Source link
Servers computers
GME Stock: DRS to ComputerShare Why It Should Matter to You
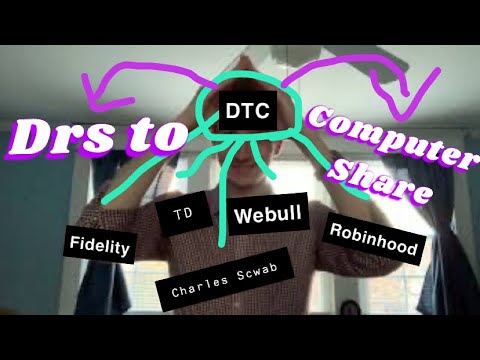
This video goes over why ComputerShare matters and what it actually means to register your shares in your own name. All in all, just providing my GME update and documenting the journey of my own personal investment in GameStop.
–
This channel does NOT provide financial advice. I do not provide financial advice. Only sharing my thoughts and opinions, always do your own research!
–
Instagram:
Tweets by TetronInvest
Twitter:
Tweets by TetronInvest
source
Technology
Numa raises $32M to bring AI and automation to car dealerships
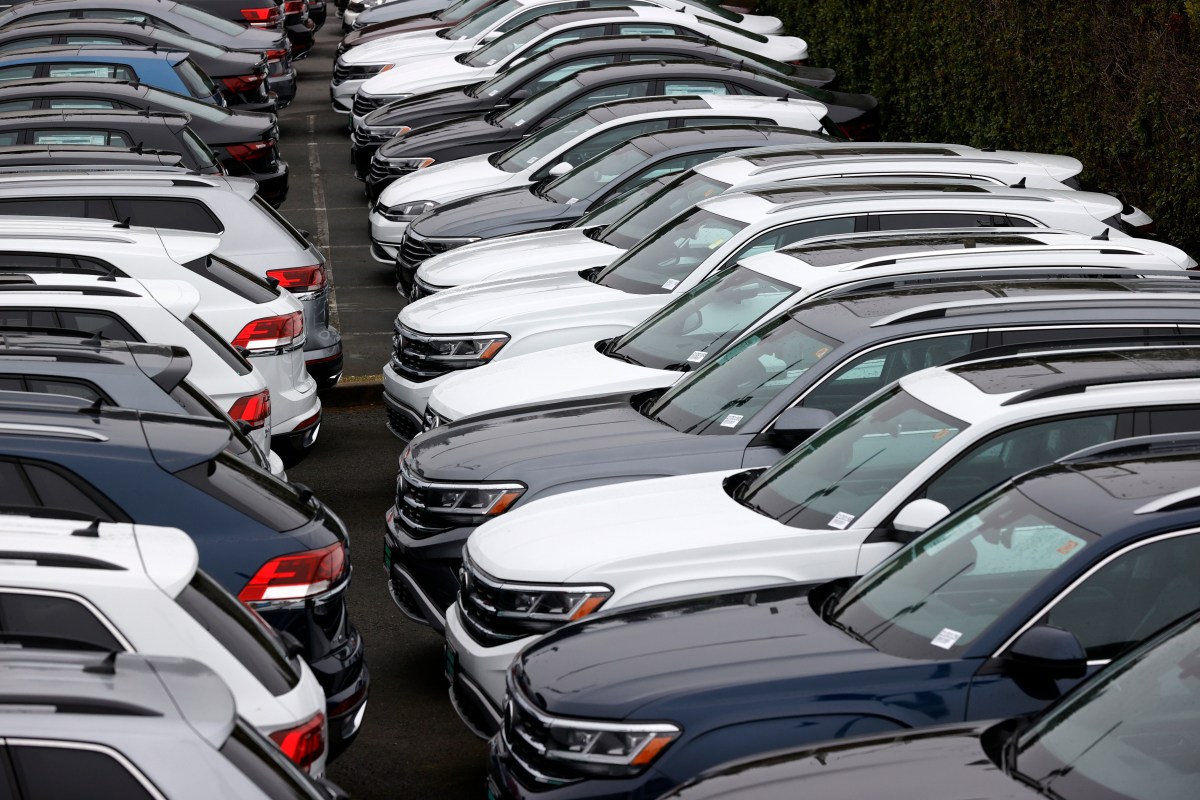
Sometimes, a pivot ends up being the smartest decision company leaders can make. See Netflix’s pivot from DVDs to streaming, or Corning’s pivot from lightbulbs to touchscreens.
The list of extremely successful startup pivots goes on. And on. And on.
A less-prominent (but by no means failed) pivot is Numa’s. Its co-founders killed the startup’s original conversational AI product to instead sell customer service automation tools. Not just any tools, though — these tools are targeted at auto dealerships.
That sounds like a highly specific niche, but it’s been profitable, according to Tasso Roumeliotis, Numa’s CEO. The company closed a $32 million Series B round in September.
“We were early to build AI and conversational commerce,” Roumeliotis told TechCrunch in an interview. “But we decided to focus our AI entirely on the automotive vertical after identifying enormous opportunity in that space.”
Roumeliotis co-founded Numa in 2017 with Andy Ruff, Joel Grossman, and Steven Ginn. Grossman hails from Microsoft, where he helped ship headliner products like Windows XP, as well as a few less recognizable ones like MSN Explorer. Ruff, another Microsoft veteran, led the team that created the first Outlook for Mac client.
Numa is actually the co-founders’ second venture together. Roumeliotis, Grossman, Ginn, and Ruff previously started Location Labs, a family-focused security company that AVG bought for $220 million 10 years ago.
What rallied the old crew behind Numa, Roumeliotis says, was a shared belief in the potential of “thoughtfully applied” AI to transform entire industries. “The market is full of AI and automation point solutions or broad, unfocused tools,” he said. “Numa offers an end-to-end solution that prioritizes the needs of the customer: car dealerships.”
The U.S. has more than 17,000 new-car dealerships, representing a $1.2 trillion industry. Yet many dealerships struggle to manage customer service requests. Per one survey, a third of dealers miss at least a fifth of their incoming calls.
Poor responsiveness leads to low customer service scores, which in turn hurt sales. But Numa can prevent things from getting that bad — or so Roumeliotis claims — by tackling the low-hanging fruit.

Numa uses AI to automate tasks such as “rescuing” missed calls and booking service appointments. For example, if a customer rings a dealership but hangs up immediately afterward, Numa can send a follow-up text or automatically place a reminder call. The platform can also give customers status updates on ongoing service, and facilitate trade-ins by collecting any necessary information ahead of time.
“Many dealerships still rely on legacy systems that are inefficient and lack integration with modern, AI-driven platforms,” Roumeliotis said. “Today’s consumers expect fast, seamless interactions across all platforms. Dealerships struggle to meet these expectations, especially in areas like real-time communication, service updates, and personalized experiences, which AI can help address.”
Other small-time automation vendors (e.g., Brooke.ai, Stella AI) provide products designed to ease dealerships’ customer service burdens. Tech giants, meanwhile, sell a range of generic solutions to automate away customer service. But Roumeliotis argues that Numa stands out because it understands how workflows within dealerships impact the end-customer experience.
“Dealership service leaders and employees are running around constantly, handling customers in person, going out to check on cars and parts, dealing with ringing phones, and balancing coordination with co-workers,” Roumeliotis said. “Numa brings that all together in a way intentionally designed with AI and the user inside the dealership to drive how the platform works rather than the other way around.”
Roumeliotis asserts Numa has another advantage in its in-house models, which drive the platform’s automations. He said the models were trained on datasets from OEMs and dealership systems as well as conversation data between dealerships and clients.
Were each one of these clients, OEMs, and dealerships informed that their data would be used to train Numa’s models? Roumeliotis declined to say. “Numa’s models are bootstrapped by a feedback loop between dealerships, customers interacting with dealerships, and the usage of Numa to facilitate this,” he said.

That answer probably won’t satisfy privacy-conscious folk, but it’s seemingly immaterial to many dealerships. Numa has 600 customers across the U.S. and Canada, including the largest retail auto dealership in the world. Roumeliotis claims Numa is “just about” cash-flow break-even.
“We don’t need capital to continue scaling revenue,” he added. “Instead, Numa is using its money to accelerate product development by expanding our team of AI and machine learning engineers, including investing in building AI models for the automotive vertical.” The company currently has 70 employees.
Benefiting Numa in its conquest is the willingness of dealerships to pilot AI to abstract away certain back-office work.
According to a survey by automotive software provider CDK Global last year, 67% of dealerships are using AI to identify sales leads, while 63% have deployed it for service. Those responding to the poll were quite bullish on the tech overall, with close to two-thirds saying that they anticipated positive returns.
Touring Capital and Mitsui, a Japanese conglomerate that’s one of the largest shareholders in automaker Penske, led Numa’s Series B round. Costanoa Ventures, Threshold Ventures, and Gradient, Google’s AI-focused venture fund, also participated in the round. The funding brings Oakland-based Numa’s total raised to $48 million.
Servers computers
Full Form of Computer 💻 ||
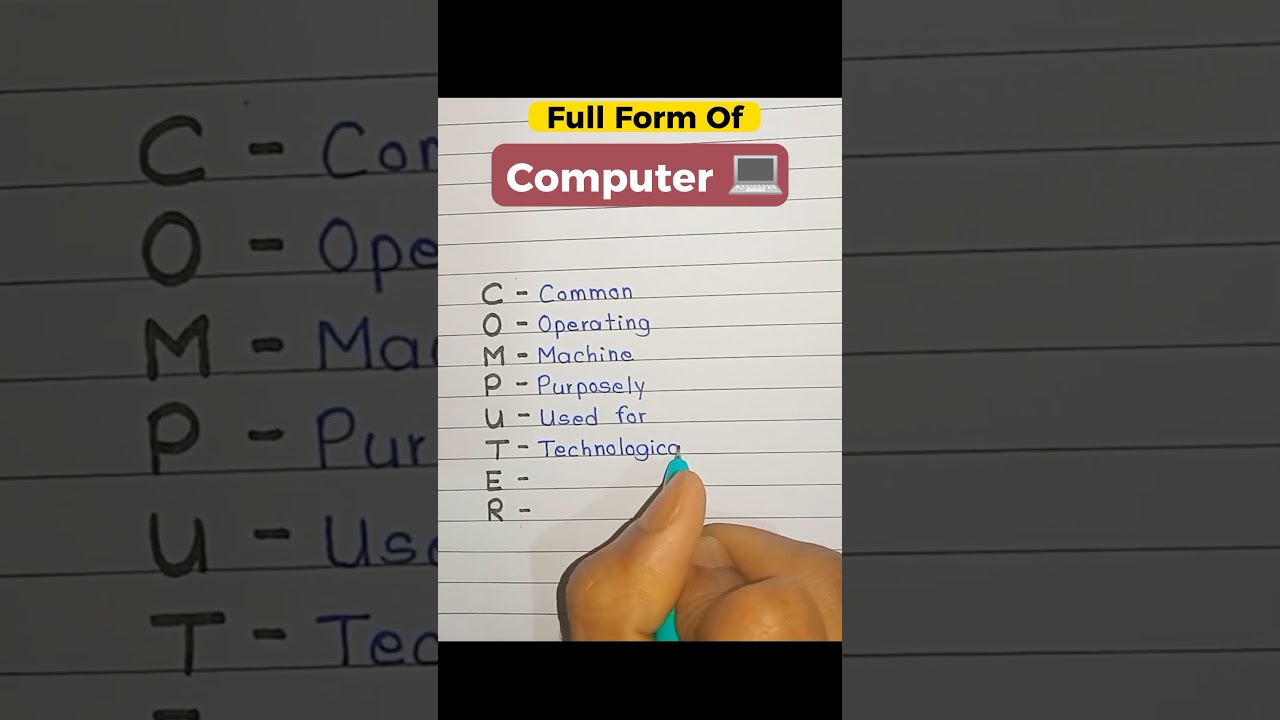
Full Form of Computer 💻 ||
.
.
.
.
.
.
.
.
.
.
.
.
#computer
#fullform
#computerfullform
#computerfullforms
#gk
#gkshorts
#generalknowledge
#important
#importantfullform
#study
#studymotivation
#educational
#educationalvideo
#laptop
#computerfullform .
source
Technology
JD Vance avoids January 6th questions by pivoting to Facebook ‘censorship’
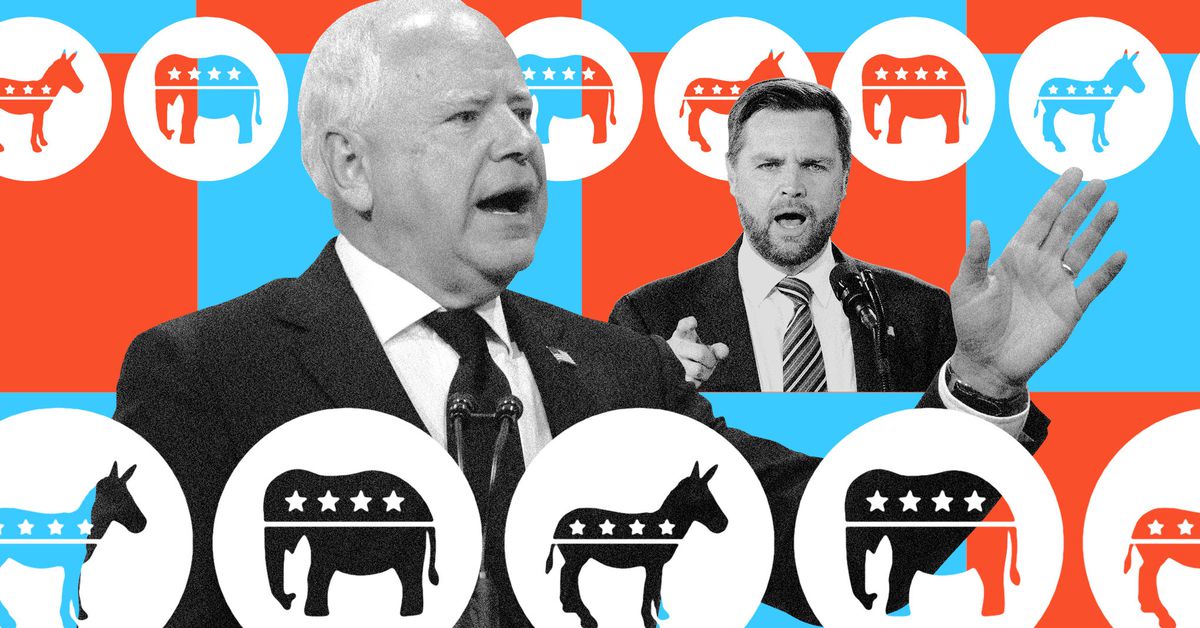
“You have said you would not have certified the last presidential election, and would have asked the states to submit alternative electors. That has been called unconstitutional and illegal,” moderator Norah O’Donnell asked Vance. “Would you again seek to challenge this year’s election results, even if every governor certifies the results?”
Vance said that instead of the threats to democracy decried by Democrats, what’s really worrying is the threat of “big technology companies silencing their fellow citizens.” Vance says Harris would like to “censor people who engage in misinformation,” and that’s “a much bigger threat to democracy than anything we’ve seen” in the last four or 40 years.
“Kamala Harris is engaged in censorship at an industrial scale,” Vance said, adding that’s a much larger threat than former President Donald Trump telling people to protest “peacefully” on January 6th at the US Capitol insurrection. Vance compared Trump’s refusal to believe the results of the 2020 election to Democrats’ concerns about Russian foreign interference in the 2016 election, where they pointed to foreign agents’ purchasing of Facebook ads as contributing to Hillary Clinton’s loss to Trump. (A Republican-led Senate committee concluded in 2020 that Russia did seek to interfere in the 2016 election to benefit Trump’s candidacy.)
“January 6th was not Facebook ads,” Walz retorted, calling Vance’s version of events “revisionist history.”
“January 6th was not Facebook ads”
Vance was apparently alluding to the events behind Murthy v. Missouri, a Supreme Court case decided earlier this year. The case covered accusations that the Biden administration coerced tech platforms to engage in censorship. Justices ruled in the Biden administration’s favor based on standing, but they also cast doubt on whether there was a meaningful connection between government outreach to platforms like Facebook and those platforms’ later moderation decisions.
Walz attempted to redirect the debate back to the original question. “Did he lose the 2020 election?” he asked Vance.
“Tim, I’m focused on the future,” Vance replied. “Did Kamala Harris censor Americans from speaking their mind in the wake of the 2020 Covid situation?”
“That is a damning non-answer,” Walz said.
“It’s a damning non-answer for you not to talk about censorship,” Vance retorted.
At another point, Vance accused Harris of wanting to “use the power of government and Big Tech to silence people from speaking their minds.” Trump himself recently suggested that some people “should be put in jail the way they talk about our judges and our justices,” referring to criticism of the Supreme Court.
Walz responded to Vance with the widely used but misleading claim that “shouting fire in a crowded theatre” is a Supreme Court test for unprotected speech. Vance didn’t dispute the premise, but he claimed “you guys wanted to kick people off of Facebook for saying that toddlers shouldn’t wear masks. That’s not fire in a crowded theatre. That is criticizing the policies of the government, which is the right of every American.”
“I don’t run Facebook,” Walz said. “This is not a debate, it’s not anything anywhere other than in Donald Trump’s world.”
-
Womens Workouts1 week ago
3 Day Full Body Women’s Dumbbell Only Workout
-
Technology2 weeks ago
Would-be reality TV contestants ‘not looking real’
-
Science & Environment2 weeks ago
Hyperelastic gel is one of the stretchiest materials known to science
-
Science & Environment2 weeks ago
‘Running of the bulls’ festival crowds move like charged particles
-
Science & Environment2 weeks ago
Maxwell’s demon charges quantum batteries inside of a quantum computer
-
Science & Environment2 weeks ago
How to wrap your mind around the real multiverse
-
News1 week ago
Our millionaire neighbour blocks us from using public footpath & screams at us in street.. it’s like living in a WARZONE – WordupNews
-
Science & Environment2 weeks ago
Sunlight-trapping device can generate temperatures over 1000°C
-
Science & Environment2 weeks ago
How to unsnarl a tangle of threads, according to physics
-
Science & Environment2 weeks ago
ITER: Is the world’s biggest fusion experiment dead after new delay to 2035?
-
Science & Environment2 weeks ago
Liquid crystals could improve quantum communication devices
-
Science & Environment2 weeks ago
Physicists are grappling with their own reproducibility crisis
-
Science & Environment2 weeks ago
Quantum ‘supersolid’ matter stirred using magnets
-
News2 weeks ago
You’re a Hypocrite, And So Am I
-
Sport2 weeks ago
Joshua vs Dubois: Chris Eubank Jr says ‘AJ’ could beat Tyson Fury and any other heavyweight in the world
-
Science & Environment2 weeks ago
Quantum forces used to automatically assemble tiny device
-
Science & Environment2 weeks ago
Why this is a golden age for life to thrive across the universe
-
Science & Environment2 weeks ago
Nuclear fusion experiment overcomes two key operating hurdles
-
Science & Environment2 weeks ago
Caroline Ellison aims to duck prison sentence for role in FTX collapse
-
Science & Environment2 weeks ago
Time travel sci-fi novel is a rip-roaringly good thought experiment
-
Science & Environment2 weeks ago
Laser helps turn an electron into a coil of mass and charge
-
News2 weeks ago
Israel strikes Lebanese targets as Hizbollah chief warns of ‘red lines’ crossed
-
Science & Environment2 weeks ago
Nerve fibres in the brain could generate quantum entanglement
-
CryptoCurrency2 weeks ago
Cardano founder to meet Argentina president Javier Milei
-
Science & Environment1 week ago
Meet the world's first female male model | 7.30
-
Womens Workouts2 weeks ago
Best Exercises if You Want to Build a Great Physique
-
CryptoCurrency2 weeks ago
Ethereum is a 'contrarian bet' into 2025, says Bitwise exec
-
News2 weeks ago
▶️ Media Bias: How They Spin Attack on Hezbollah and Ignore the Reality
-
Science & Environment2 weeks ago
Why we need to invoke philosophy to judge bizarre concepts in science
-
CryptoCurrency2 weeks ago
Bitcoin miners steamrolled after electricity thefts, exchange ‘closure’ scam: Asia Express
-
CryptoCurrency2 weeks ago
DZ Bank partners with Boerse Stuttgart for crypto trading
-
CryptoCurrency2 weeks ago
Bitcoin bulls target $64K BTC price hurdle as US stocks eye new record
-
Womens Workouts2 weeks ago
Everything a Beginner Needs to Know About Squatting
-
News1 week ago
Four dead & 18 injured in horror mass shooting with victims ‘caught in crossfire’ as cops hunt multiple gunmen
-
Womens Workouts1 week ago
3 Day Full Body Toning Workout for Women
-
Travel1 week ago
Delta signs codeshare agreement with SAS
-
Politics7 days ago
Hope, finally? Keir Starmer’s first conference in power – podcast | News
-
Sport2 weeks ago
UFC Edmonton fight card revealed, including Brandon Moreno vs. Amir Albazi headliner
-
Technology2 weeks ago
iPhone 15 Pro Max Camera Review: Depth and Reach
-
News2 weeks ago
Brian Tyree Henry on voicing young Megatron, his love for villain roles
-
Science & Environment2 weeks ago
A slight curve helps rocks make the biggest splash
-
Science & Environment2 weeks ago
Quantum time travel: The experiment to ‘send a particle into the past’
-
CryptoCurrency2 weeks ago
Dorsey’s ‘marketplace of algorithms’ could fix social media… so why hasn’t it?
-
CryptoCurrency2 weeks ago
RedStone integrates first oracle price feeds on TON blockchain
-
CryptoCurrency2 weeks ago
Low users, sex predators kill Korean metaverses, 3AC sues Terra: Asia Express
-
CryptoCurrency2 weeks ago
Blockdaemon mulls 2026 IPO: Report
-
CryptoCurrency2 weeks ago
Coinbase’s cbBTC surges to third-largest wrapped BTC token in just one week
-
News1 week ago
Why Is Everyone Excited About These Smart Insoles?
-
Science & Environment2 weeks ago
A new kind of experiment at the Large Hadron Collider could unravel quantum reality
-
Science & Environment2 weeks ago
How one theory ties together everything we know about the universe
-
Science & Environment2 weeks ago
Being in two places at once could make a quantum battery charge faster
-
CryptoCurrency2 weeks ago
Crypto scammers orchestrate massive hack on X but barely made $8K
-
Science & Environment2 weeks ago
Tiny magnet could help measure gravity on the quantum scale
-
Science & Environment2 weeks ago
Future of fusion: How the UK’s JET reactor paved the way for ITER
-
Science & Environment2 weeks ago
How do you recycle a nuclear fusion reactor? We’re about to find out
-
CryptoCurrency2 weeks ago
Telegram bot Banana Gun’s users drained of over $1.9M
-
CryptoCurrency2 weeks ago
VonMises bought 60 CryptoPunks in a month before the price spiked: NFT Collector
-
CryptoCurrency2 weeks ago
SEC asks court for four months to produce documents for Coinbase
-
CryptoCurrency2 weeks ago
‘No matter how bad it gets, there’s a lot going on with NFTs’: 24 Hours of Art, NFT Creator
-
Business2 weeks ago
How Labour donor’s largesse tarnished government’s squeaky clean image
-
News2 weeks ago
Brian Tyree Henry on voicing young Megatron, his love for villain roles
-
Womens Workouts2 weeks ago
How Heat Affects Your Body During Exercise
-
Womens Workouts2 weeks ago
Keep Your Goals on Track This Season
-
News2 weeks ago
Church same-sex split affecting bishop appointments
-
Technology2 weeks ago
Fivetran targets data security by adding Hybrid Deployment
-
Politics2 weeks ago
Labour MP urges UK government to nationalise Grangemouth refinery
-
Health & fitness2 weeks ago
The maps that could hold the secret to curing cancer
-
News2 weeks ago
the pick of new debut fiction
-
CryptoCurrency2 weeks ago
$12.1M fraud suspect with ‘new face’ arrested, crypto scam boiler rooms busted: Asia Express
-
Science & Environment2 weeks ago
UK spurns European invitation to join ITER nuclear fusion project
-
CryptoCurrency2 weeks ago
Decentraland X account hacked, phishing scam targets MANA airdrop
-
CryptoCurrency2 weeks ago
CertiK Ventures discloses $45M investment plan to boost Web3
-
CryptoCurrency2 weeks ago
Beat crypto airdrop bots, Illuvium’s new features coming, PGA Tour Rise: Web3 Gamer
-
CryptoCurrency2 weeks ago
‘Silly’ to shade Ethereum, the ‘Microsoft of blockchains’ — Bitwise exec
-
CryptoCurrency2 weeks ago
Vitalik tells Ethereum L2s ‘Stage 1 or GTFO’ — Who makes the cut?
-
CryptoCurrency2 weeks ago
Ethereum falls to new 42-month low vs. Bitcoin — Bottom or more pain ahead?
-
Business2 weeks ago
Thames Water seeks extension on debt terms to avoid renationalisation
-
Politics2 weeks ago
‘Appalling’ rows over Sue Gray must stop, senior ministers say | Sue Gray
-
News2 weeks ago
Brian Tyree Henry on his love for playing villains ahead of “Transformers One” release
-
Womens Workouts2 weeks ago
Which Squat Load Position is Right For You?
-
Science & Environment1 week ago
CNN TÜRK – 🔴 Canlı Yayın ᴴᴰ – Canlı TV izle
-
Technology1 week ago
Robo-tuna reveals how foldable fins help the speedy fish manoeuvre
-
News5 days ago
US Newspapers Diluting Democratic Discourse with Political Bias
-
Technology2 weeks ago
Is carbon capture an efficient way to tackle CO2?
-
Politics2 weeks ago
Trump says he will meet with Indian Prime Minister Narendra Modi next week
-
Science & Environment2 weeks ago
Single atoms captured morphing into quantum waves in startling image
-
Technology2 weeks ago
Can technology fix the ‘broken’ concert ticketing system?
-
Fashion Models2 weeks ago
Mixte
-
Science & Environment2 weeks ago
How Peter Higgs revealed the forces that hold the universe together
-
Health & fitness2 weeks ago
The secret to a six pack – and how to keep your washboard abs in 2022
-
CryptoCurrency2 weeks ago
2 auditors miss $27M Penpie flaw, Pythia’s ‘claim rewards’ bug: Crypto-Sec
-
CryptoCurrency2 weeks ago
Journeys: Robby Yung on Animoca’s Web3 investments, TON and the Mocaverse
-
CryptoCurrency2 weeks ago
Louisiana takes first crypto payment over Bitcoin Lightning
-
CryptoCurrency2 weeks ago
‘Everything feels like it’s going to shit’: Peter McCormack reveals new podcast
-
Science & Environment2 weeks ago
A tale of two mysteries: ghostly neutrinos and the proton decay puzzle
-
CryptoCurrency2 weeks ago
SEC sues ‘fake’ crypto exchanges in first action on pig butchering scams
-
CryptoCurrency2 weeks ago
Bitcoin price hits $62.6K as Fed 'crisis' move sparks US stocks warning
-
CryptoCurrency2 weeks ago
CZ and Binance face new lawsuit, RFK Jr suspends campaign, and more: Hodler’s Digest Aug. 18 – 24
-
Politics2 weeks ago
UK consumer confidence falls sharply amid fears of ‘painful’ budget | Economics
-
News2 weeks ago
Bangladesh Holds the World Accountable to Secure Climate Justice
You must be logged in to post a comment Login